
Figure 1: Countries’ vulnerability to climate change is highly correlated with poverty
As social scientists, we are ultimately driven by a desire to understand how societies form, and how they organise themselves as social systems. We are interested in how people live, and interact with one another. Although, we might often be interested in understanding the socio-economic dynamics of a place, whether it is a city or an entire country, the stark absence of accurate and up-to-date data on almost all metrics crucially limits us in which places we can study. How can we study, better understand, and ultimately find solutions for improving living standards of places if we are missing vital information?
Additionally, it is exactly the same places that require urgent knowledge to boost economic development and lift people out of poverty that also suffer the most from absence and nonexistent data. It is also those same places that are the most vulnerable to climate change (see Figure 1). This is the ultimate motivation that kickstarted my adventure to solve this issue by combining my expertise in economics with geospatial AI and remote sensing.
Satellites sense optical and visible information that also includes those invisible to the human eye e.g. infrared and ultraviolet information. This has led to substantial advances in the understanding of processes across space for multiple domains, including crop and harvest surveillance, or disaster related building destruction analyses. Although, in the context of socioeconomic dynamics, we are often looking for information that is inherently “invisible”. For example, you cannot “see” poverty, unemployment, or political attitudes from a satellite. However, while poverty itself is not a “visible” phenomenon, we can observe information from satellites that we know to be related to poverty. For instance, we can separate houses into shacks and mansions, we can see which areas are lit up at night and which are dark, and we can see roads that tell us which places are well connected to economic hubs and which places are very spatially remote.
Merging domain knowledge from the social sciences with an understanding of remote sensing and geospatial AI is thus a powerful combination that can generate pioneering insights that are urgently needed to answer some of the most burning questions for policymakers. Domain knowledge allows us to identify the most important questions concerning societies. Without this contextual knowledge we would struggle to understand the complex information that we retrieve as data. In fact, we might misinterpret the data and thus end up building models that are meaningless or even harmful. Remote sensing and geospatial AI on the other hand allow us to better understand places where the absence of traditional data wouldn’t generally permit this. They also allow us to geographically expand our analyses so that we can generate insights robustly and at scale. Together, domain knowledge, computing power and remote sensing is a powerful asset.
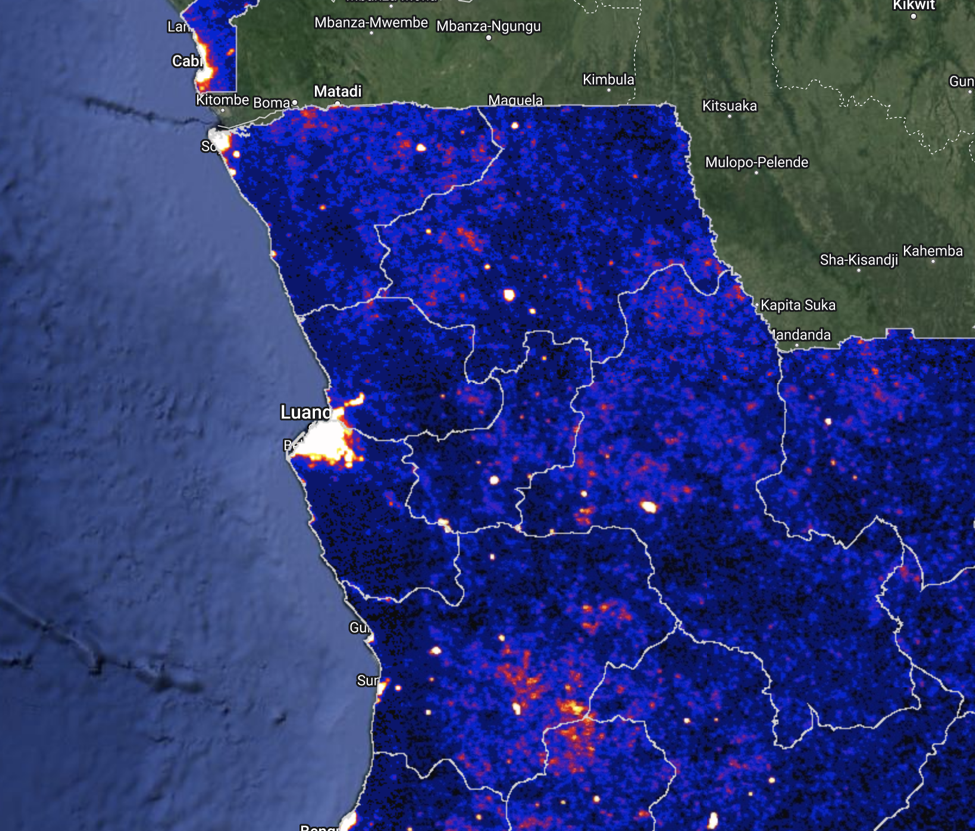
Socio-economic mapping on the basis of EO has gained some attention in recent years. Night lights have been established as a common form of understanding GDP dynamics when measured GDP is absent or unreliably measured (see Figure 2). More recently, the socio-economic discussion also includes pioneering work on mapping poverty is for example provided by Yeh et al. (2022) where deep learning (DL) algorithms have been trained on publicly available satellite imagery to better understand village level wealth across a large sample of African countries. Another example is from my own research, where I have built algorithms on the basis of EO data and OpenStreetMap information to predict spatial urban employment patterns within cities across developing countries. This work supports the World Bank across projects in the context of sustainable urbanisation and urban climate hazards with urgently missing and more precise information on the spatial organisation of cities (you can find the paper here, figure 3 below refers to this work).
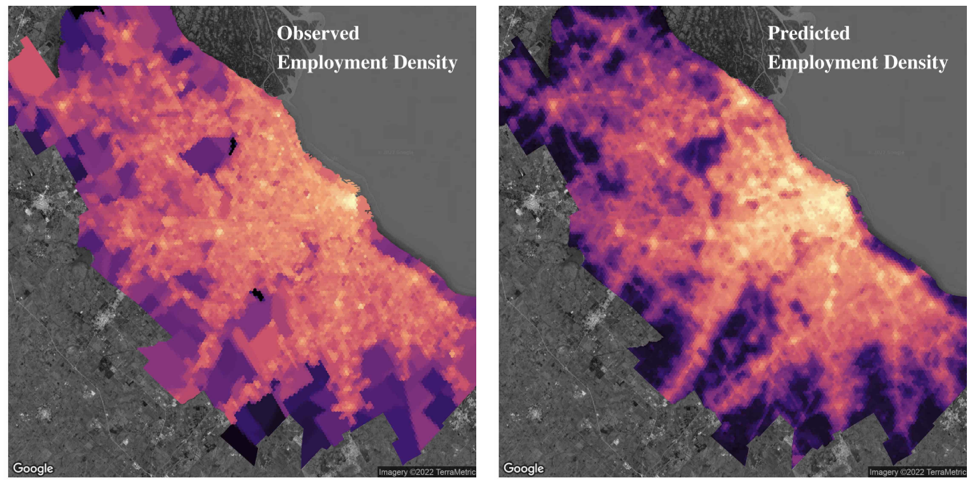
Figure 3: Predicted vs. ground truth/observed employment patterns across Buenos Aires (Source: Barzin et al., 2022)
Satellite and remote sensing allow us to better understand our societies. The applications are powerful and plentiful, ranging from capturing poverty patterns and documenting human rights abuses in war zones, to allowing people to prevent food crises by monitoring food security and understanding where emergency aid is most needed after disasters (see figure 4 below). However, important bottlenecks remain. While we have vast catalogues of imagery available, we require reliably labelled ground truth data to connect raw imagery to meaningful information. Ground truth data, however, still remains absent for many areas of the world and for many of the invisible socioeconomic variables.

Figure 4: Building Destruction Assessment after the 2023 Türkiye and Syria Earthquakes (Source: MIT Technology Review)
Overall, without domain knowledge and careful ethical awareness that the data that we model will affect peoples’ lives, there remains a risk that these technological advances may do more harm than good. Satellites, Earth Observation (EO), and geospatial AI in their essence are technological advances that provide us with information and data, and that is where the immense power comes from.
We must use this power to change the lives of people on this planet for a better and safer future, where less people have to live in poverty. In doing this, we need to ensure that the analysis of remotely sensed information incorporates knowledges from other domains (to ensure that we fully understand the socio-economic dynamics), and that we bridge the gaps between the geospatial innovations being produced by firms, the knowledge created on understanding our societies generated by academics, and the questions policymakers face. Ultimately ensuring that geospatial AI contributes to making our planet a better place for generations to come.
About the Author
Samira Barzin (PhD) is a Senior Researcher at the University of Oxford. Samira works on combining Earth Observation (EO), big data and geospatial AI specifically for policy questions on economic development in countries of the global south. Samira is particularly focussed on highlighting the substantial role EO can play for better knowledge and more effective policy solutions to lift people out of poverty and address some of the most urgent questions on sustainable economic development in the era of climate change.
Find out how Samira Barzin has worked with the World Bank to use data analysis techniques to inform development interventions:
Commenti